HEALTH POLICY AND MANAGEMENT RESEARCH
Researchers Create AI Algorithm to Improve Timeliness, Accuracy of Sepsis Predictions
November 25, 2021 ·
Contributed by: The Research Institute of St. Joe's Hamilton
Share
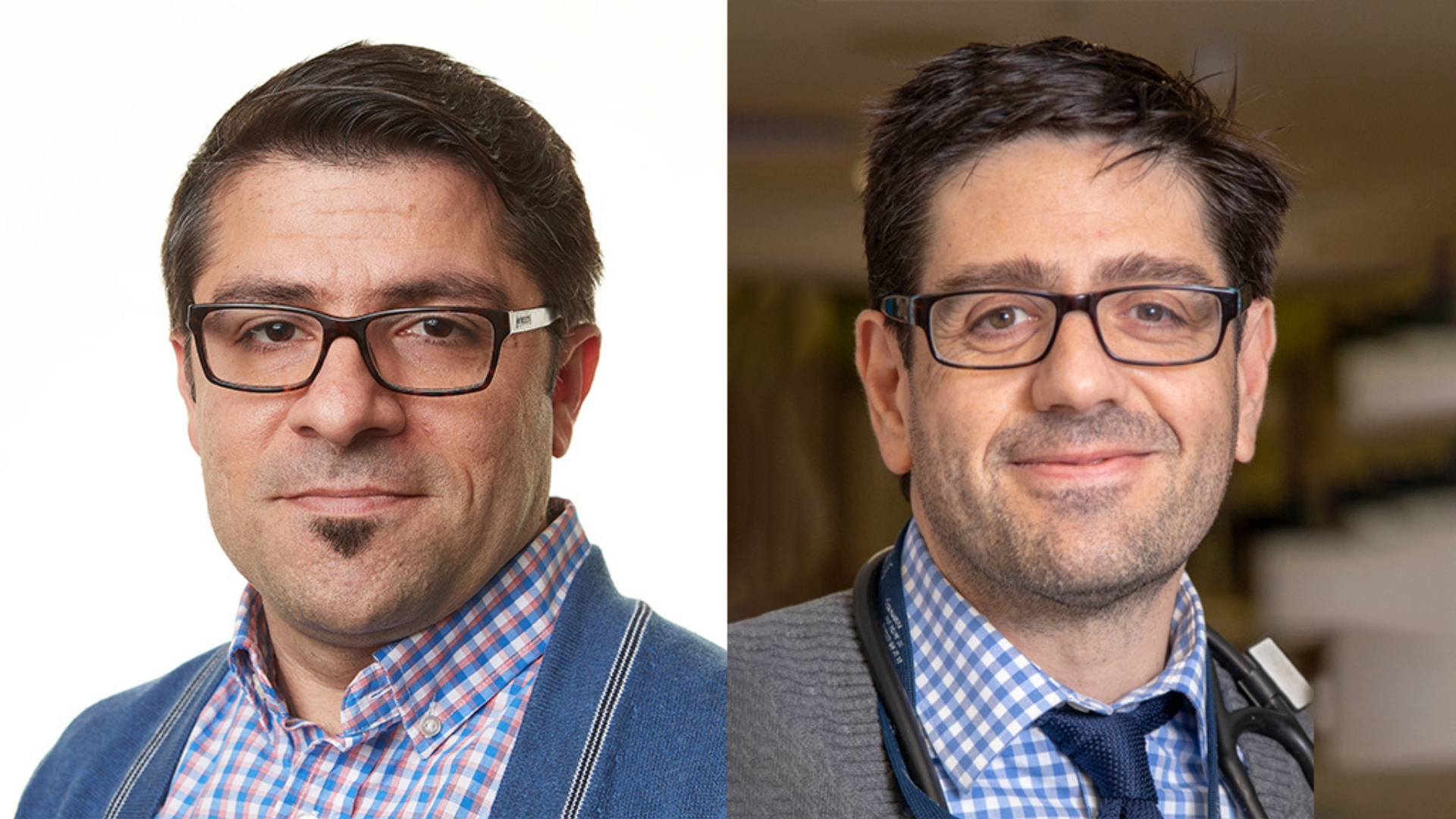
Each year, sepsis affects more than 30 million people worldwide, causing an estimated six million deaths. Sepsis is the body’s extreme response to an infection and is often life-threatening.
Since every hour of delayed treatment can increase the odds of death by four to eight per cent, timely and accurate predictions of sepsis are crucial to reduce morbidity and mortality. To that end, various health care organizations have deployed predictive analytics to help identify patients with sepsis by using electronic medical record (EMR) data.
An international research team, including data scientists, physicians, and engineers from McMaster University and St. Joseph’s Healthcare Hamilton, have created an Artificial Intelligence (AI) predictive algorithm that greatly improves the timeliness and accuracy of data-driven sepsis predictions.
“Sepsis can be predicted very accurately and very early using AI with clinical data, but the key questions to the clinician and data scientists are how much historical data these algorithms need to make accurate predictions and how far ahead they can predict sepsis accurately,” said Manaf Zargoush, study co-author and assistant professor of health policy and management at McMaster’s DeGroote School of Business.
To predict sepsis in clinical care settings, some systems use EMR data with disease scoring tools to determine sepsis risk scores – essentially acting as digital, automated assessment tools. More advanced systems employ predictive analytics, such as AI algorithms, to go beyond risk assessment and identify sepsis itself.
Using AI predictive analytics, researchers created an algorithm called the Bidirectional Long Short-Term Memory (BiLSTM). It examines several variables across four key domains: administrative variables (e.g., length of the Intensive Care Unit (ICU) stay, hours between hospital and ICU admission, etc.), vital signs (e.g., heart rate and pulse oximetry, etc.), demographics (e.g., age and gender), and laboratory tests (e.g., serum glucose, creatinine, platelet count, etc.). Compared to other algorithms, the BiLSTM is a more complex subset of machine learning – called deep learning – that uses neural networks to increase its predictive power.
The study compared the BiLSTM with six other machine learning algorithms and found it was superior to the others in terms of accuracy. Improving accuracy by reducing false positives is key to a successful algorithm, since these errors not only waste medical resources, but they also erode physicians’ confidence in the algorithm.
Interestingly, the study found that predictive accuracy may be increased through algorithms that focus more heavily on a patient’s recent datapoints, instead of looking back further to include as many datapoints as possible.
Researchers noted that it is understandable that clinicians would be inclined to populate the algorithm with as many data points as possible over a long timeframe. However, their findings suggest that when the purpose of prediction is being accurate and timely regarding sepsis predictions, physicians with long prediction horizons should rely more on the fewer yet more recent clinical data of the patient.
“St. Joe’s will be launching a cognitive computing pilot project in late November that includes understanding how AI can be used to help predict sepsis in real patients and in real time,” said Dan Perri, study co-author, physician, and chief information officer at St. Joseph’s Healthcare Hamilton. He is also an associate professor of medicine at McMaster.
“Understanding the breadth and scope of data that enables sepsis prediction is important for any organization looking at using AI to save lives from severe infections,” Perri added.
“Learnings from sepsis models translate into building better machine learning tools that lead to appropriate early intervention for some of the sickest patients, while also avoiding unnecessary warnings that could lead to health care worker fatigue.”
The study was published in the journal Nature Scientific Reports.